Author | Affiliation |
---|---|
David J. Kim, MD | The Warren Alpert Medical School of Brown University, Department of Emergency Medicine, Providence, Rhode Island |
Esther K. Choo, MD, MPH | The Warren Alpert Medical School of Brown University, Department of Emergency Medicine, Providence, Rhode Island |
Megan L. Ranney, MD, MPH | The Warren Alpert Medical School of Brown University, Department of Emergency Medicine, Providence, Rhode Island |
Introduction
Methods
Results
Discussion
Limitations
Conclusion
ABSTRACT
Introduction:
Technology-based interventions offer an opportunity to address high-risk behaviors in the emergency department (ED). Prior studies suggest behavioral health strategies are more effective when gender differences are considered. However, the role of gender in ED patient preferences for technology-based interventions has not been examined. The objective was to assess whether patient preferences for technology-based interventions varies by gender.
Methods:
This was a secondary analysis of data from a systematic survey of adult (≥18 years of age), English-speaking patients in a large urban academic ED. Subjects were randomly selected during a purposive sample of shifts. The iPad survey included questions on access to technology, preferences for receiving health information, and demographics. We defined “technology-based” as web, text message, e-mail, social networking, or DVD; “non-technology-based” was defined as in-person, written materials, or landline. We calculated descriptive statistics and used univariate tests to compare men and women. Gender-stratified multivariable logistic regression models were used to examine associations between other demographic factors (age, race, ethnicity, income) and technology-based preferences for information on specific risky behaviors.
Results:
Of 417 participants, 45.1% were male. There were no significant demographic differences between men and women. Women were more likely to use computers (90.8% versus 81.9%; p=0.03), Internet (66.8% versus 59.0%; p=0.03), and social networks (53.3% versus 42.6%; p=0.01). 89% of men and 90% of women preferred technology-based formats for at least type of health information; interest in technology-based for individual health topics did not vary by gender. Concern about confidentiality was the most common barrier to technology-based use for both genders. Multivariate analysis showed that for smoking, depression, drug/alcohol use, and injury prevention, gender modified the relationship between other demographic factors and preference for technology-based health information; e.g., older age decreases interest in technology-based information for smoking cessation in women but not in men (aOR 0.96, 95% CI 0.93-0.99 versus aOR 1.00, 95% CI 0.97-1.03).
Conclusion:
Our findings suggest ED patients’ gender may affect technology preferences. Receptivity to technology-based interventions may be a complex interaction between gender and other demographic factors. Considering gender may help target ED patient populations most likely to be receptive to technology-based interventions.
INTRODUCTION
The utility of preventative care in reducing healthcare utilization and promoting healthy behaviors has been well established.1–4 Research has repeatedly demonstrated that even a brief intervention by a healthcare provider can result in behavior change or serve as a bridge to further intervention, presenting a unique opportunity for emergency providers.1,2,5–7 Emergency departments (ED) routinely encounter a wide range of high risk, potentially preventable behaviors, including substance abuse, violence, high-risk sexual practices, and untreated mental health conditions, often from patients who use the ED as their only connection to healthcare.3 Time constraints, cost, and competing clinical priorities have historically deterred many providers from offering these needed services in the ED setting, increasing morbidity for patients and the cost to the health system as a whole.3,8 The potential for technology-based interventions has piqued the interest of many ED physicians as a feasible, efficient, cost-effective solution to provide these needed services out of the ED.6,9,10
Gender has been shown to play a significant role in both the likelihood of high-risk behaviors and in responsiveness to interventions for these high-risk behaviors.11–15 Multiple studies have suggested that multidimensional targeting to specific subpopulations, taking gender into consideration, increases responsiveness, prevents miscommunication, and enhances the likelihood of resulting in behavioral change.16–21 Gender also seems to play a significant role in attitudes towards technology-based health information.22–25 For example, men are more likely than women to be overall users of the Internet, but use online content more for leisure, including gaming, or for commercial or financial transactions; women have been shown to be more purpose-driven and more engaged with online health information.22,26
Previous studies have examined overall patient responsiveness to technology-based interventions for high-risk behaviors,27,28 but few data exist on the effect of gender on ED patients’ preferences toward these interventions or the interaction between gender and other demographic considerations when gauging interest in technology-based health information. The objective of this secondary analysis was to perform a gender-stratified examination of the preferences of adult ED patients towards a technology-based intervention.
METHODS
This was a secondary analysis of a previously published cross sectional survey study of English-speaking adult (18 years or age or older) patients on preferences for technology-based health information.27Participants were recruited from a high volume (approximately 105,000 annual visits) urban, tertiary care ED in New England. The ED serves a population that is approximately 60% white, 20% black, and 20% Hispanic, with 30% receiving government assistance. The institutional review board of the participating hospital approved all study procedures of the original survey study.
Research assistants (RAs) screened and enrolled a random sample of adult patients presenting to the ED for care, covering a convenience sample of shifts between the hours of 7AM to 12AM, 7 days a week, during a 9-month period. A computerized random number generator directed the RAs to rooms of patients to be screened for eligibility.
Patients were eligible for the study if they were clinically stable (Emergency Severity Index 2 to 5 as defined by the Agency for Healthcare Research and Quality29 or as determined by the attending physician) and literate in English. Exclusion criteria included: being unable to provide verbal consent (presenting intoxicated), presenting with a psychiatric chief complaint, a victim of sexual assault, in police custody, or self-reporting previous completion of the survey. The data were made anonymous, and responses were not reported to clinical providers.
The survey collected information from eligible patients on demographics (including age, gender, education, and income), baseline technology use, preferences for receiving health-related information on 7 pre-defined risky behaviors through a series of technology-based modalities, and concerns for receiving health information through various technology mediums (full survey online at http://www.annemergmed.com, Appendix E2, E3).27 We defined a “technology-based” intervention as web, text message, email, social networking, or DVD; “non-technology-based” was defined as being delivered in-person, written materials, or landline. The 7 pre-defined risky behaviors for the survey were: unintentional injury, peer violence, interpersonal violence, mental health, smoking, alcohol/substance abuse, and risky sex. We chose the topics based on the critical public health areas outlined by the 2009 Society for Academic Emergency Medicine Consensus Conference, “Public Health in the Emergency Department: Surveillance, Screening, & Intervention.”5 Questions regarding baseline technology-based use and behavioral intervention preferences were adapted, when possible, from validated questions administered to previous populations.23,28,30,32 Demographic questions were from the Behavioral Risk Factor Surveillance System.33Participants completed the survey on an iPad, using DatStat (DatStat Illume, Seattle, WA), a HIPAA-compliant Web-based survey technology. If patients expressed discomfort using the iPad, they were permitted to complete the survey on paper; research assistants entered any paper survey data immediately into DatStat.
We calculated descriptive statistics (mean, standard deviations) and univariate comparisons (t-tests for continuous variables and Chi-squared tests for categorical variables) for demographic characteristics, current technology use, risky behaviors, intervention preferences, and concerns about technology-based behavioral health interventions. We also used univariate comparisons, including 95% confidence intervals (CIs), to calculate gender differences in these variables.
We used gender-stratified multivariate logistic regression models to examine associations between other demographic factors (age, race, ethnicity, income) and intervention preferences (e.g., preferring technology- vs. non-technology based interventions). The goals of this analysis were to: 1) analyze whether demographic factors correlated differentially with a patient’s intervention preference for the 2 genders; and 2) examine whether the influence of gender on a patient’s preferences for and concerns about technology-based interventions varied based on the health topic. We defined the reference variables for the analyses were defined as: age (entered as continuous variable), race (white), ethnicity (Non-Hispanic), and income (low; below poverty line defined as income <$25,000 or receiving public assistance). All statistical analyses were conducted using Stata 10 SE (Stata Corp LP, College Station, TX).
RESULTS
A total 993 adult patients were randomly chosen for screening. Of those screened, 69.2% were eligible, and of those eligible, 60.7% (417 patients) consented to participate and completed the survey (Figure); 45.1% of those consented were male. There were no significant demographic differences between male and female patients (Table 1). No information is available regarding unscreened patients; there were no significant gender differences between eligible patients who consented and refused for the study. Thirty-six patients (8.7%) completed paper questionnaires, and demographics of this group were similar to those completing surveys on iPads.
At baseline, women were more likely than men to use computers (90.8% versus 81.9%; p=0.03), Internet (66.8% versus 59.0%; p=0.03), and social networks (53.3% versus 42.6%; p=0.01). Most participants – 89.4 % of men and 90.8% of women – preferred a technology format for at least 1 type of health information. In the univariate analysis, there were no significant gender differences in preferred format for the various intervention topics.
Confidentiality was the most common concern about technology-based use for both genders across all mediums. A larger percentage of women expressed concern about confidentiality than men regarding social networking interventions (38.9% versus 26.6%, p=0.008). Males expressed significantly greater concerns about accessing health information by cell phone than women (4.8% versus 0.9%, p=0.01). There were no other significant differences between men and women regarding barriers to technology-based interventions.
Gender-stratified multivariable analysis showed that for smoking, depression, drug/alcohol use, and injury prevention, gender modified the relationship between other demographic factors and preference for technology-based health information based on the specific health topic (Table 2). For example, when asked about peer violence interventions, higher income increased women’s odds of preferring a technology-based intervention, but income had no relationship with technology-based intervention for men. For information about depression, both younger men and women were more interested in information through a technology-based medium, but ethnicity only played a role for men and not for women.
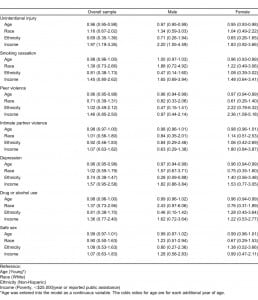
Multivariate analysis of preference for technology-based health information (adjusted odd ratios with 95% confidence interval).
For all significant independent variables, the overall odds ratio was the approximate mean of the stratified odds ratios, consistent with an interaction effect of gender with that variable. In the case of peer violence, the unstratified odds ratio for income obscured the effect of income on receptivity to technology-based information among women.
DISCUSSION
Several previous studies have examined the impact of gender on engagement with technology-based health information with most finding that females are more likely to be engaged than males.17,24,26,34 Previous studies have found that the impact of gender on the desire to receive health information varies in relationship to other demographic variables.35–39 This study supports this finding, specifically for preferences for technology-based interventions, and underscores the potential insight offered by a gender-stratified approach.
For instance, we found that younger age increases women’s preferences for receiving information on smoking cessation through a technology-based medium, while age did not affect men’s preferences for technology for that topic. In this case, an unstratified analysis for smoking cessation intervention preferences did not reflect the interaction between gender and age.40 Similarly, the unstratified analysis did not indicate that income level was a factor in preference for technology-based information on peer violence; a stratified analysis, however, reveals a relationship between higher income and technology preference in women only. The findings of this study may help providers more specifically identify and categorize subpopulations that prefer technology-based interventions, and others that might find non technology-based programs more acceptable.
Unlike previous studies, this study also highlights gender differences in preferences for technology-based mediums in the ED population. In an environment where time and resource constraints often prevent individual targeting of interventions, our findings suggest that using a gender-specific approach may help enhance utility of available resources by identifying subpopulations that may be most receptive.
Considering gender, and identifying target subpopulations more open to technology-based interventions, may be particularly useful for groups with historic disparities in access to care. For example, several previous studies have demonstrated that men are less likely than women to seek treatment for depression.19,41 Our study’s data suggest that young, non-Hispanic males may be more receptive towards receiving information about depression through a technology-based medium, thereby identifying a potential avenue to intervene for a subpopulation that may have been previously undertreated. It is important to note that, as an exploratory study, this analysis did not match interest in a behavioral intervention with assessments of that specific behavior; assessing interest in a broad range of technologies within groups demonstrating high-risk behaviors is still incompletely studied.
LIMITATIONS
The strength of this study is the use of a systematic survey that included validated questions whenever possible. Stratifying by gender allowed us to examine the relationship between gender and other demographic variables and interest in technology-based health information in a way not possible by including gender as a single independent variable. However, there were also study limitations. The study was conducted in 1 urban academic ED in the Northeast with only English-speaking adults. The attitudes in this population may not be generalizable to other populations with different demographics, education levels, and societal norms. The survey was primarily conducted on an iPad interface, which may have introduced a social desirability bias towards technology-based mediums. However, participants were given the option to complete the survey on paper to minimize any effect, and the demographics from those that elected to complete the survey on iPad are consistent with those that elected to complete the survey on paper. Any potential social desirability impact on preferences are likely to be consistent among both men and women and unlikely to affect any findings regarding gender differences in preferences, which was the focus of this secondary analysis. This analysis was also limited to exploring gender differences in relationship to age, race, ethnicity, and income level. Future studies may want to include additional variables, such as educational level, as an expanded analysis may reveal additional important insights into patient preferences for technology-based information. As a secondary data analysis, we may be underpowered to detect differences between genders that are present (i.e. Type II error); the relatively large confidence intervals in Table 2 reflect the small sample size of this study. Several of the identified differences produced a confidence interval approximating the value of one. The significance of these differences may change in a larger study that includes more participants. Finally, it is important to note that this analysis explored gender differences in interest in interventions through a technology-based medium. It does not explore gender differences in overall interest for health information regarding these health topics. It also did not explore gender differences in acceptability or feasibility of technology-based interventions.
CONCLUSION
Gender plays a major role in initiating and continuing high-risk health behaviors; our study suggests it may also play a role in preferences for technology-based versus traditional interventions to address these types of behaviors in the ED. A gender-specific approach to designing behavioral interventions may result in screening and intervention strategies that are more acceptable and effective. Future studies will be needed to determine how using a gender-specific framework can optimize the effectiveness of brief, technology-based interventions in the ED population.
Footnotes
Supervising Section Editor: Abigail Hankin, MD, MPH
Full text available through open access at http://escholarship.org/uc/uciem_westjem
Conflicts of Interest: By the WestJEM article submission agreement, all authors are required to disclose all affiliations, funding sources and financial or management relationships that could be perceived as potential sources of bias. The authors disclosed none.